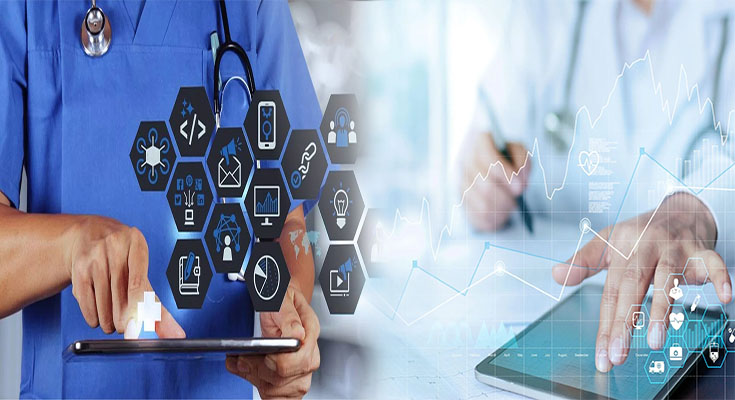
Blockchain Technology for Secure and Transparent Healthcare Data Management
In recent years, the healthcare industry has been exploring innovative technologies for better managing and securing patient data. One such technology that holds great promise is blockchain. With its decentralized and transparent nature, blockchain has the potential to revolutionize healthcare data management, providing secure and reliable solutions for storing and sharing sensitive information.
What is Blockchain Technology?
Blockchain technology is a decentralized and distributed ledger that records and verifies transactions across multiple computers. It relies on cryptographic algorithms to ensure the security and integrity of data stored within the network. Unlike traditional centralized systems, where data is stored and controlled by a single entity, blockchain allows for a transparent and tamper-proof record of transactions.
The Need for Secure Healthcare Data Management
Healthcare data is incredibly valuable and sensitive. It includes patient medical records, personal information, and financial data. Protecting this information from unauthorized access, tampering, and breaches is crucial to …
Blockchain Technology for Secure and Transparent Healthcare Data Management Read More